Federated Learning
Smart Homes: Knowledge Repository
Energy usage data is routinely sent to energy companies for purposes such as billing and demand forecasting. However, this time-series data can reveal far more than just energy consumption – it can inadvertently expose sensitive information about household activities and behaviours, leading to significant privacy concerns. The charts below illustrate some of the potential risks associated with leaking such data. As you review the patterns in these charts, try to identify possible threats that could arise if this information falls into the wrong hands.
placeholder
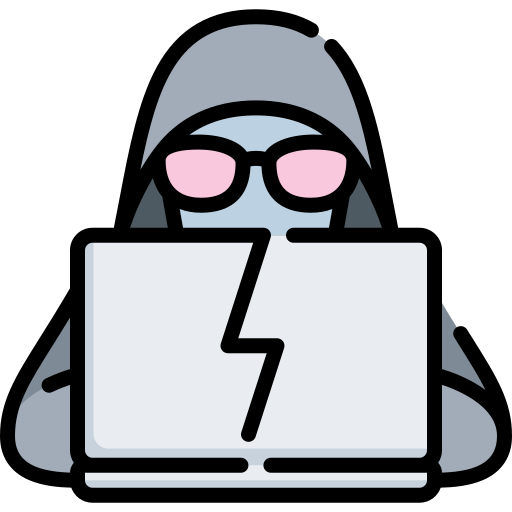
The charts above highlight major privacy threats associated with the leakage of raw energy consumption data, emphasising the need for privacy-preserving solutions. To address these concerns, federated learning (FL) provides a novel approach that prevents raw data from being transmitted to energy companies. Instead, only model parameters are shared, ensuring that sensitive household information remains private.
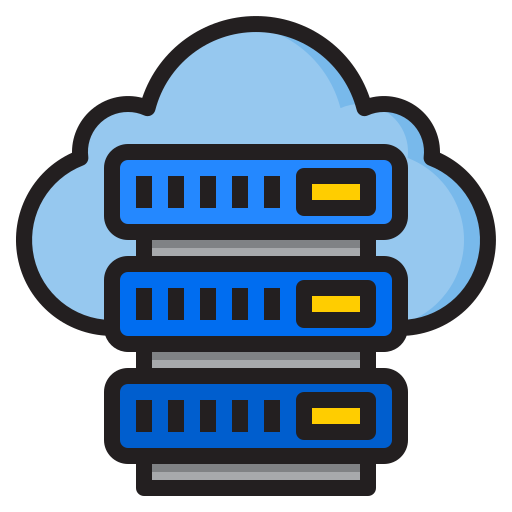
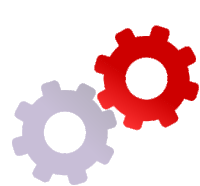
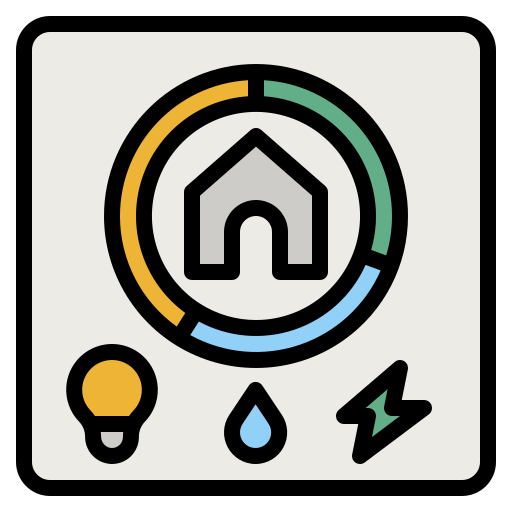
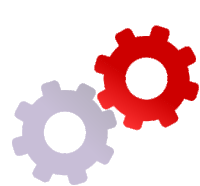
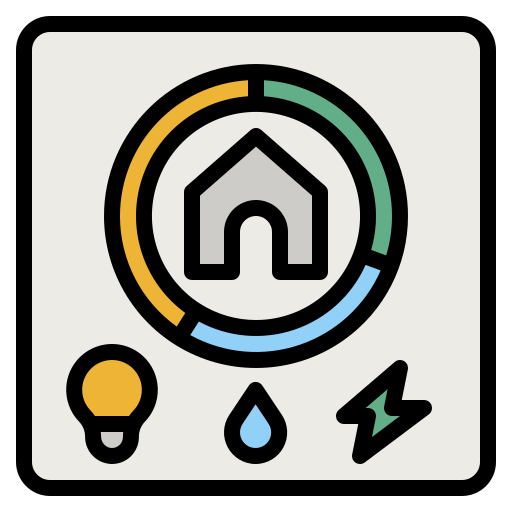
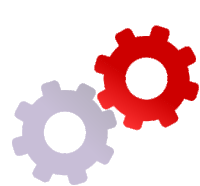
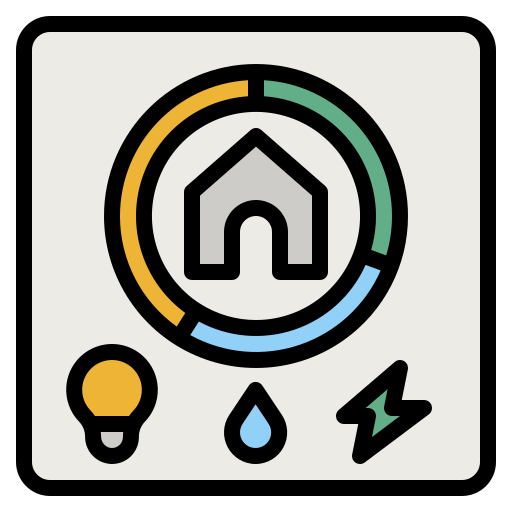
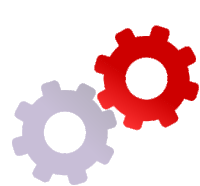
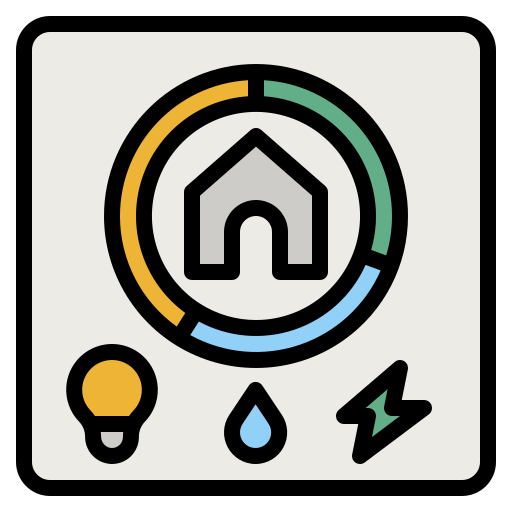
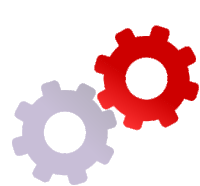
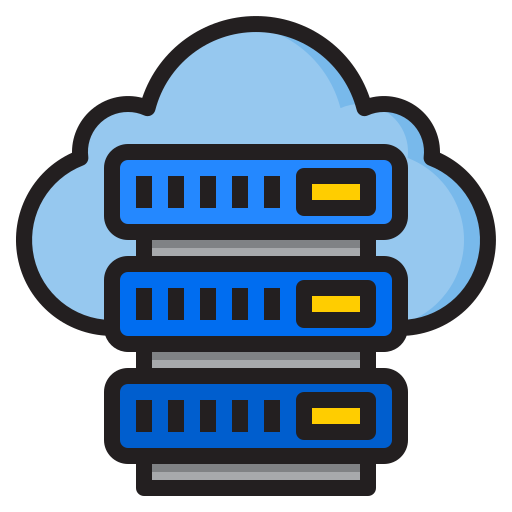
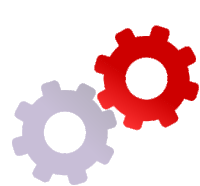
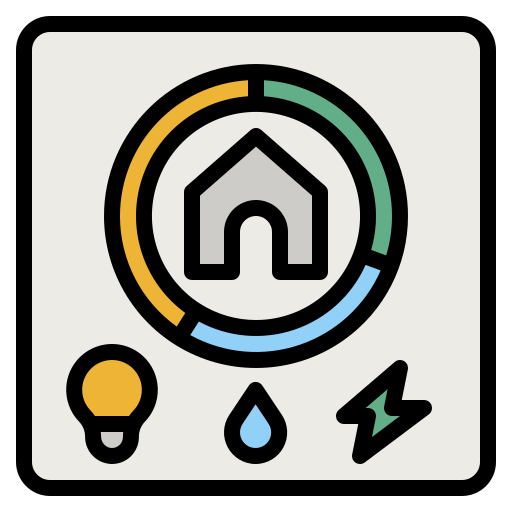
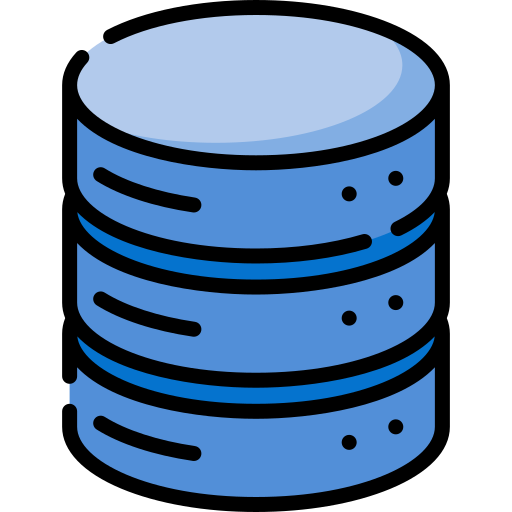
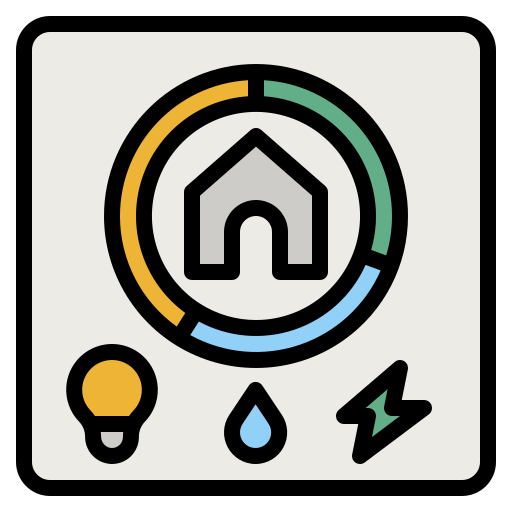
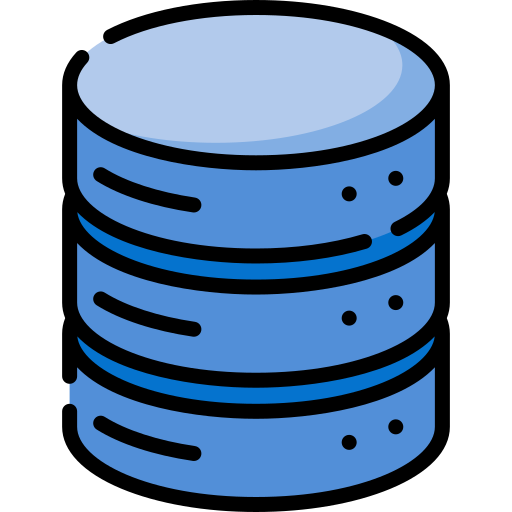
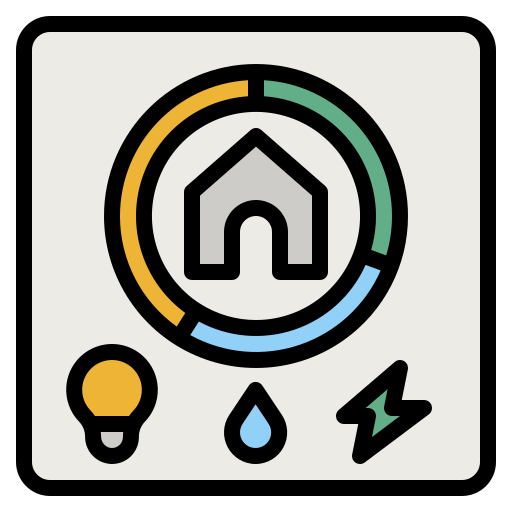
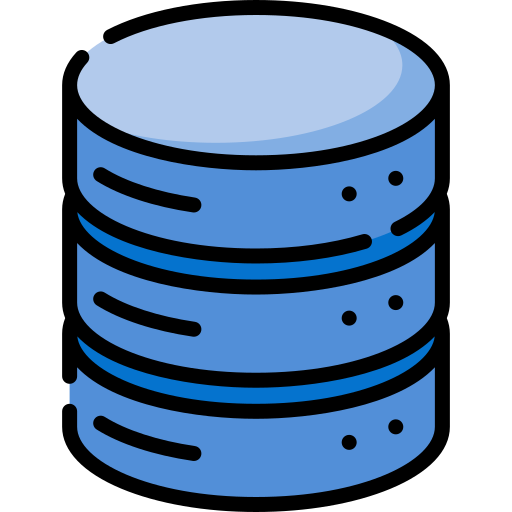
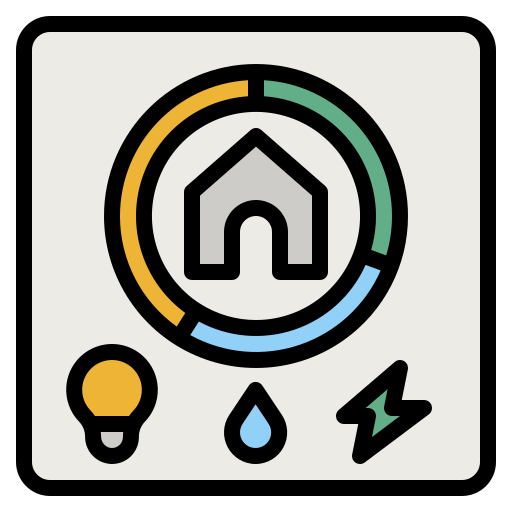
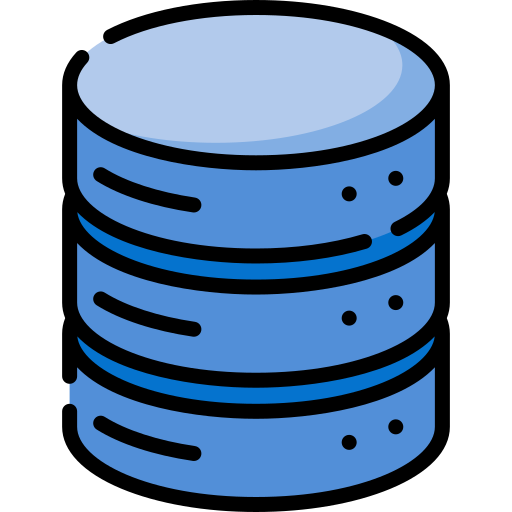
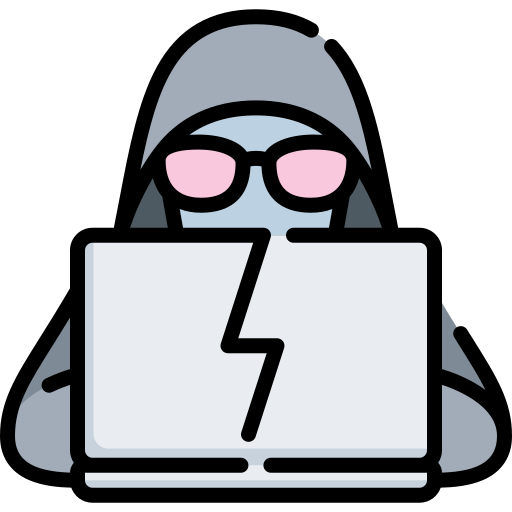
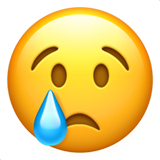
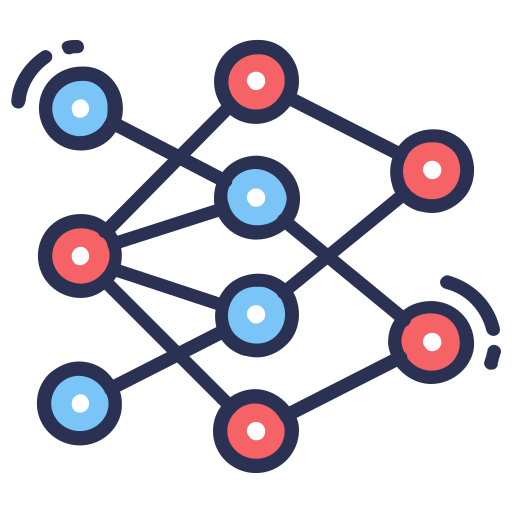
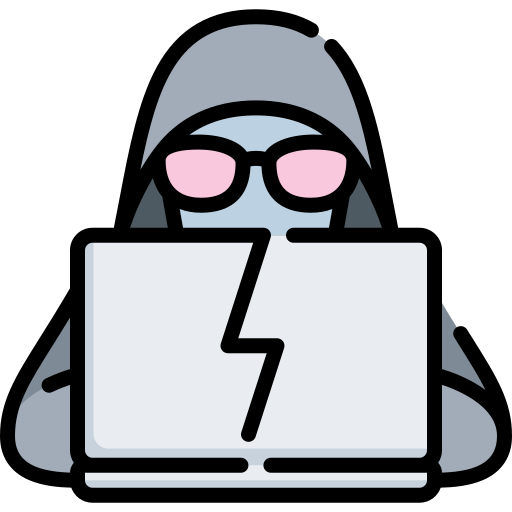
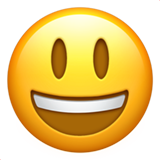
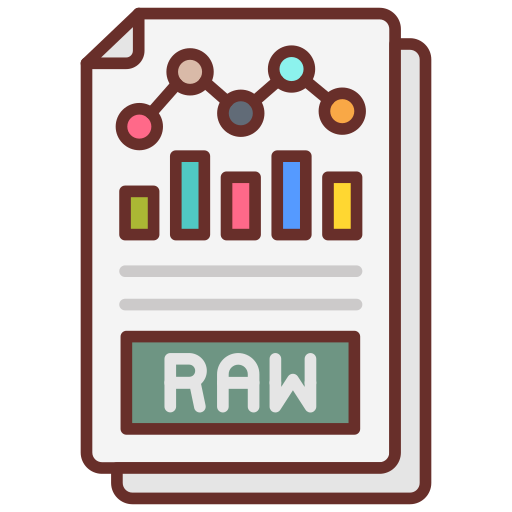
To demonstrate the effectiveness of this approach, we conducted a computer simulation experiment using energy consumption forecasting as a case study. Our findings revealed that FL not only mitigates privacy risks but also improves prediction accuracy over local training and centralised training. This enhanced performance arises from the generalisation ability of federated models, which leverage insights from multiple local models to better capture diverse consumption patterns. These results underscore the potential of FL to provide both robust privacy protection and superior forecasting capabilities.
For detailed information on the technical implementation, experiment design, and analysis results, please refer to our paper presented at the 8th IEEE Conference on Energy Internet and Energy System Integration (IEEE EI² 2024).